
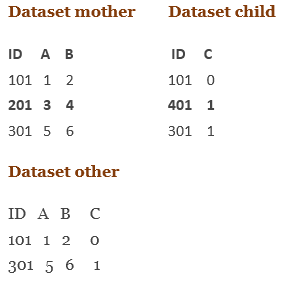
The chained equation approach to multiple imputation In contrast, if the observed data are highly predictive of the missing values the imputations will be more consistent across imputations, resulting in smaller, but still accurate, standard errors (Greenland and Finkle, 1995). On a simple level, if there is not much information in the observed data (used in the imputation model) regarding the missing values, the imputations will be very variable, leading to high standard errors in the analyses. Because multiple imputation involves creating multiple predictions for each missing value, the analyses of multiply imputed data take into account the uncertainty in the imputations and yield accurate standard errors. Multiple imputation procedures, particularly MICE, are very flexible and can be used in a broad range of settings. Described in detail by Schafer and Graham ( 2002), the missing values are imputed based on the observed values for a given individual and the relations observed in the data for other participants, assuming the observed variables are included in the imputation model. Multiple imputation involves filling in the missing values multiple times, creating multiple “complete” datasets. Multiple imputation has a number of advantages over these other missing data approaches.
MERGE DATASETS IN SAS INTERVIEW QUESTIONS SOFTWARE
Maximum likelihood methods are sometimes a viable approach for dealing with missing data (Graham, 2009) however, these methods are primarily available only for certain types of models, such as longitudinal or structural equation models, and can generally be run only using special software such as Amos (SPSS, 2009a) and Lisrel (Scientific Software International, 2006). This will lead to overly precise results and the potential for incorrect conclusions. Single imputation procedures, such as mean imputation, are an improvement but do not account for the uncertainty in the imputations once the imputation is completed, analyses proceed as if the imputed values were the known, true values rather than imputed.

While complete case analysis may be easy to implement it relies upon stronger missing data assumptions than multiple imputation and it can result in biased estimates and a reduction in power (Graham, 2009). In practice, these circumstances rarely occur. when there is less than 5% missingness and the missingness is totally random and does not depend on observed or unobserved values), complete case analysis may be an acceptable approach to addressing missing data (Graham, 2009 Schafer, 1999). There are many different approaches to addressing missing data and the first question researchers might ask is “why use multiple imputation?” In certain circumstances (e.g. Readers interested in learning about other methods of addressing missing data can refer to Graham ( 2009), Lee and Carlin ( 2010), or Schafer ( 1999). A brief review of software available to implement MICE procedures and to analyze data that has been imputed using these procedures is also provided. This paper provides an introduction to implementing MICE with a focus on the practical aspects. However, despite these benefits, many psychiatric researchers have not yet learned about this approach, there are few practical resources available to assist in its implementation and until recently software limitations inhibited general researchers and practitioners from using the MICE procedure.
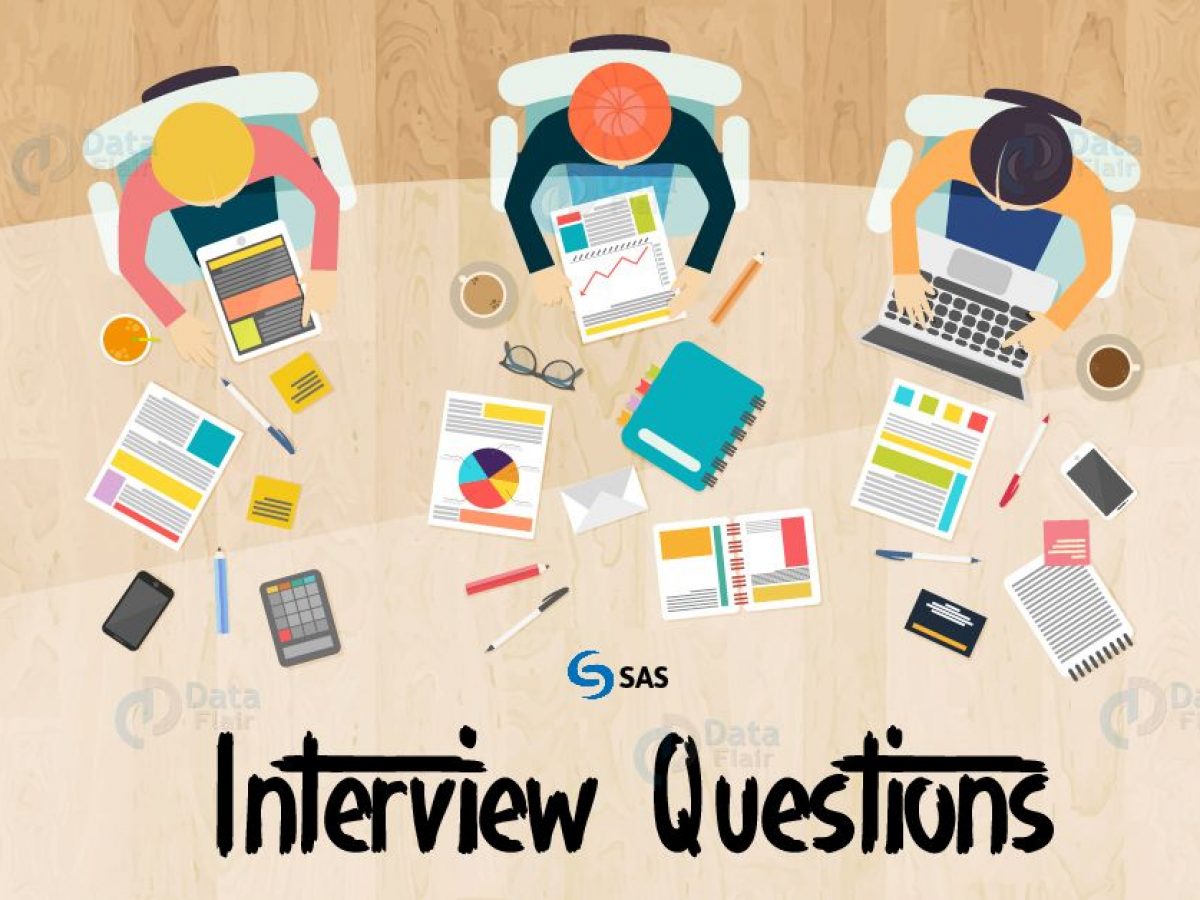
continuous or binary) as well as complexities such as bounds or survey skip patterns. In addition, the chained equations approach is very flexible and can handle variables of varying types (e.g.
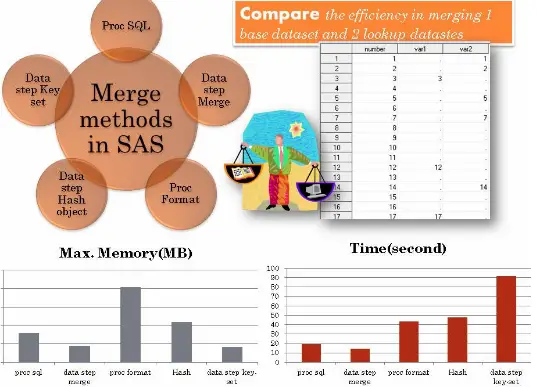
Creating multiple imputations, as opposed to single imputations, accounts for the statistical uncertainty in the imputations. Multivariate imputation by chained equations (MICE), sometimes called “fully conditional specification” or “sequential regression multiple imputation” has emerged in the statistical literature as one principled method of addressing missing data. Missing data are a common problem in psychiatric research.
